人工智能研究者获得诺贝尔物理学奖
文摘
2024-10-09 14:13
美国
英国经济学人网站于2024年10月8日发表题为《人工智能研究者获得诺贝尔物理学奖》的文章。以下观点不代表任何译者立场,现将全文翻译如下:Science & technology | The 2024 Nobel prizesAI researchers receive the Nobel prize for physicsThe award, to Geoffrey Hinton and John Hopfield, stretches the definition of the field此次颁奖给杰弗里·辛顿和约翰·霍普菲尔德,拓展了物理学领域的定义photograph: aip emilio segrè visual archiveNobel science prizes are awarded in three areas: physics, chemistry and physiology or medicine. But occasionally some noteworthy discovery comes along that does not really fit into any of them. In 1973, for example, three pioneering students of animal behaviour, who worked on honeybees, geese and sticklebacks, were shoehorned into the physiology category.诺贝尔科学奖通常授予物理、化学和生理或医学三个领域。然而,有时会出现一些不完全符合这些类别的重大发现。例如,1973年,三位研究蜜蜂、鹅和刺鱼行为的开创性动物学家被勉强归入生理学类别。Similar flexibility, though in an area with far more profound consequences than ethology, has been demonstrated with regard to this year’s physics prize. Showing a sense of timeliness not always apparent in its deliberations, Sweden’s Royal Academy of Science has stretched the definition of physics to include computer science, and given its imprimatur to two of the progenitors of the artificial-intelligence (ai) revolution that is currently sweeping all before it.今年的物理学奖展现了类似的灵活性,但涉及的领域影响远比动物行为学深远。瑞典皇家科学院表现出不同寻常的与时俱进,将物理学的定义扩展到计算机科学,并授予两位人工智能先驱这一殊荣,肯定了他们在当前席卷一切的人工智能浪潮中的贡献。John Hopfield of Princeton University and Geoffrey Hinton of the University of Toronto both did their crucial work in the early 1980s, at a time when computer hardware was unable to take full advantage of it. Dr Hopfield was responsible for what has become known as the Hopfield network—a type of artificial neural network that behaves like a physical structure called a spin glass, which gave the academy a tenuous reason to call the field “physics”. Dr Hinton’s contribution was to use an algorithm known as backpropagation to train neural networks.普林斯顿大学的约翰·霍普菲尔德和多伦多大学的杰弗里·辛顿都在20世纪80年代初完成了他们的关键研究,那时的计算机硬件还无法充分利用他们的成果。霍普菲尔德因发明了以其名字命名的霍普菲尔德网络而闻名,这是一种人工神经网络,其行为类似于自旋玻璃这种物理结构,这给了科学院将该领域归类为“物理学”的牵强理由。辛顿的贡献则是使用反向传播算法来训练神经网络。Artificial neural networks are computer programs based loosely on the way in which real, biological networks of nerve cells are believed to work. In particular, the strengths of the connections (known as weights) between “nodes” (the equivalent of neurons) in such networks are plastic. This plasticity grants a network the ability to process information differently in response to past performance; or, in other words, to learn. Hopfield networks, in which each node is connected to every other except itself, are particularly good at learning to extract patterns from sparse or noisy data.人工神经网络是基于生物神经元网络工作原理的计算机程序。特别是,这些网络中“节点”(相当于神经元)之间连接的强度(称为权重)是可塑的。这种可塑性赋予网络根据过往表现以不同方式处理信息的能力,换句话说,就是学习能力。霍普菲尔德网络中,每个节点与除自身外的所有其他节点相连,特别擅长从稀疏或噪声数据中提取模式。Dr Hinton’s algorithm turbocharged neural networks’ learning ability by letting them work, in effect, in three dimensions. Hopfield networks and their ilk are, in essence, two-dimensional. Though they actually exist only as simulations in software, they can be thought of as physical layers of nodes. Stack such layers on top of one another, though, and train them by tweaking the weights as signals move both backward and forward between the layers (ie, back-propagated as well as forward-propagated) and you have a much more sophisticated learning system.辛顿的算法通过允许网络实际上在三维空间中工作,大大提升了神经网络的学习能力。霍普菲尔德网络及其同类本质上是二维的。虽然它们实际上只是软件中的模拟,但可以被想象成物理层面的节点层。然而,将这些层叠加在一起,通过在层间来回调整权重(即反向传播和前向传播)进行训练,就形成了一个更为复杂的学习系统。Dr Hinton also, for good measure, tweaked Dr Hopfield’s networks using a branch of maths called statistical mechanics to create what are known as Boltzmann machines. (Statistical mechanics, which underlies modern understanding of the second law of thermodynamics, was invented by Ludwig Boltzmann, a near contemporary of Alfred Nobel.) Boltzmann machines can be used to create systems that learn in an unsupervised manner, spotting patterns in data without having to be explicitly taught.辛顿还利用统计力学这一数学分支改进了霍普菲尔德网络,创造了所谓的玻尔兹曼机。(统计力学是现代理解热力学第二定律的基础,由诺贝尔近乎同时期的路德维希·玻尔兹曼发明。)玻尔兹曼机可用于创建无监督学习系统,无需明确教导就能发现数据中的模式。It is, then, the activities of these two researchers which have made machine learning really sing. ai models can now not only learn, but create (or, for sceptics, reorganise and regurgitate in a most sophisticated manner). Such tools have thus gone from being able to perform highly specific tasks, such as recognising cancerous cells in pictures of tissue samples or streamlining particle-physics data, to anything from writing essays for lazy undergraduates to running robots.正是这两位研究者的成果使机器学习真正大放异彩。人工智能模型现在不仅能学习,还能创造(或者对怀疑者而言,以极其复杂的方式重组和输出)。这些工具已经从执行高度特定的任务(如识别组织样本图片中的癌细胞或简化粒子物理数据)发展到能够为懒惰的大学生写论文,甚至操控机器人。Dr Hinton, whom the academy’s detectives tracked down to a hotel in California to deliver the glad tidings, and who gamely agreed to answer questions from the press, despite the time difference, seemed both proud and worried about his achievements. He mused that by assisting mental labour, generative ai might have as big an effect on society as the industrial revolution’s assistance of physical labour has done. But he also fretted, as many in the field do, about how machine intelligence that outstripped the human variety would then go on to treat its creators. For now, though, those creators are being treated very nicely indeed. 辛顿被科学院的“侦探”们在加州一家酒店找到,得知喜讯后,尽管有时差,仍欣然同意回答记者提问。他对自己的成就既感到自豪又有所担忧。他沉思道,就像工业革命协助了体力劳动一样,生成式人工智能辅助脑力劳动可能对社会产生同样深远的影响。但他也像该领域许多人一样,对超越人类智能的机器智能将如何对待其创造者感到忧虑。不过眼下,这些创造者正受到极高的礼遇。■
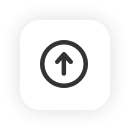