综合学术报告/11月13日
文摘
2020-11-12 18:51
题目: Nonconvex Optimization Methods for Robust Tensor Completion from Grossly Sparse Observations时间地点:11月13日下午2:00-4:00,理科楼A304 (线上报告, 腾讯会议ID:161 163 404, PW:1113)Abstract. In this talk, we consider the robust tensor completion problem for recovering a low-rank tensor image from limited samples and sparsely corrupted observations, especially by impulse noise. A convex relaxation of this problem is to minimize a weighted combination of tubal nuclear norm and the $\ell_1$-norm data fidelity term. However, the $\ell_1$-norm may yield biased estimators and fail to achieve the best estimation performance. To overcome this disadvantage, we propose and develop a nonconvex model, which minimizes a weighted combination of tubal nuclear norm, the $\ell_1$-norm data fidelity term, and a concave smooth correction term. Further, we present a Gauss-Seidel-Difference-of-Convex Algorithm (GS-DCA) to solve the resulting optimization model by using a linearization technique. We prove that the iteration sequence generated by GS-DCA converges to the critical point of the proposed model.Furthermore, we propose an acceleration technique of GS-DCA to improve the convergence of the GS-DCA. Numerical experiments for color images, hyperspectral images, Magnetic Resonance imaging (MRI) images and videos demonstrate that the effectiveness of the proposed method.白敏茹,湖南大学数学学院教授,博士生导师,担任湖南省运筹学会副理事长、湖南省计算数学与应用软件学会副理事长、中国运筹学会数学优化学会理事,长期致力于最优化理论、方法及其应用研究,近年来主要从事张量优化、低秩稀疏优化及其在图像处理中的应用研究,主持国家自然科学基金面上项目和湖南省自然科学基金等项目,取得了系列研究成果,在SIAM Journal on Imaging Sciences、Inverse Problems, Journal of Optimization Theory and Applications, Computational Optimization and Applications, Journal of Global Optimization等学术期刊上发表论文近30余篇,获得2017年湖南省自然科学二等奖(排名第二)。
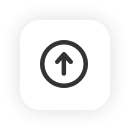